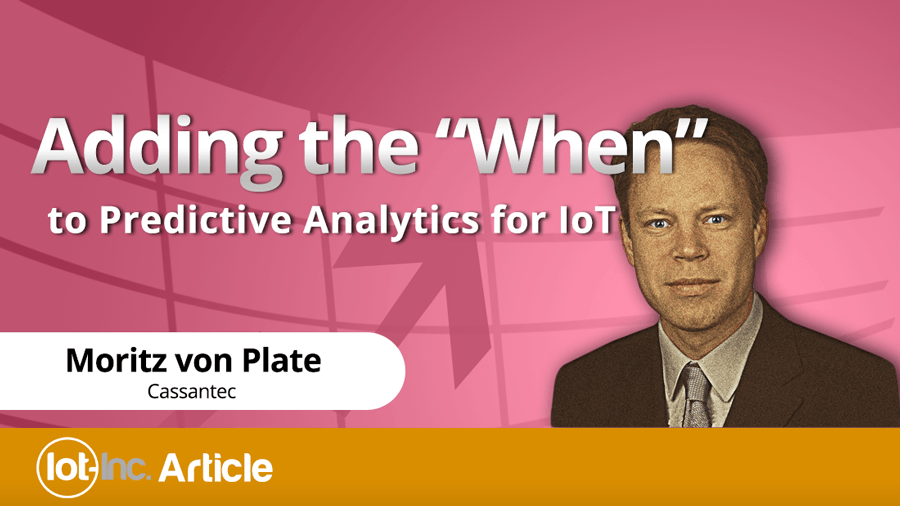
01 Mar Adding the “When” to Predictive Analytics for IoT
By Moritz von Plate
Why would you need to know then “when” in the first place? Or, what’s the purpose of Prognostics?
Many decisions around equipment deal with the future. However, in many cases these decisions are based on expert intuition and gut-feel. While these are not wrong per se, they would benefit greatly from Prognostics to reduce costly downtime, avoid unnecessary maintenance work and increase equipment availability. The financial impact on the operation is in the millions.
Many decisions around equipment deal with the future. However, in many cases these decisions are based on expert intuition and gut-feel. While these are not wrong per se, they would benefit greatly from Prognostics to reduce costly downtime, avoid unnecessary maintenance work and increase equipment availability.
With the advent of the Internet of Things (IoT) the Prognostics lever can now be pulled. Prognostics provides explicit foresight into when in the future to expect which malfunction to occur with which probability. It is based on a novel approach blending advanced data analytical methodologies and expert knowledge into a coherent, efficiently configured and scalable solution.
Other approaches are at best complementary, lacking the fundamental benefit of knowing “when”. Automated condition monitoring focuses on the current equipment condition to prevent immediate damages. Predictive Analytics, viewed by many as state-of-the-art, gets closer by providing early warnings. But, it too does not eliminate the key challenge, which is to predict not only whether but actually when a malfunction will occur. All Predictive Analytics does is to say: “Beware, malfunction XYZ is approaching!”. But when, in a day, a week or three months, remains unclear.
Hence, Prognostics is needed to close that last information gap in the IoT. Here is how to get ready for Prognostics in seven steps:
- Choosing the equipment
- Prioritizing and defining the malfunction modes
- Getting a grip on the data: define data parameters
- Correlating the data parameters to the malfunction modes
- Computing Remaining Useful Life (RUL)
- Evaluating the results
- Using the prognoses
Step 1: Choosing the equipment
The first step is to choose the mission-critical equipment. Ideally, you start with an equipment type that:
- Is critical for your plant operation;
- Is costly to bring back online in case of malfunction or damage;
- Is equipped with sensors to generate condition and process data.
Step 2: Prioritizing and defining the malfunction modes
It is important to maintain focus on the critical issues. Thus, this second step defines the top malfunction modes that – following a Pareto principle – are the main drivers of potential trouble. These could be those malfunctions that you have always managed to avoid, possibly almost literally at all cost. You could also have observed them over the last 5-10 years or have actually learned about them during a user conference.
Step 3: Getting a grip on the data: define data parameters
In parallel to step 2, you can visualize your data history to detect patterns that explain malfunctions. In many cases, though, it is not enough to look at the raw data. It is rather derivative data parameters, e.g. rates of change or synchronicity of readings, that reveal the truth. Also, since many data patterns are hard to identify, applying advanced stochastic methods will probably be required.
Questions that can help you in this step include: Can I define data diagnostic rules that could have helped detect the malfunction before it occurred? What alarm thresholds, limits and benchmarks need to be applied for detection?
Step 4: Correlating the data parameters to the malfunction modes
In step 4 correlations of data parameters to specific malfunction modes must be determined. Furthermore, a set of value ranges has to be determined for the defined parameters. A good starting point is to pull the alert and alarm thresholds from your automatic condition monitoring.
Step 5: Computing Remaining Useful Life (RUL)
In step 5 several data analytics operations need to be performed using advanced stochastic models to yield the future malfunction risk and RUL distribution for the component. Note that specialized software providers offer not only this step, but can provide an end-to-end solution supporting the move towards Prognostics across all seven steps.
Step 6: Evaluating the results
This step aims at checking for the plausibility of the results. One approach can be to conduct a retrospective analysis on prior time periods. This allows a validation with actual observations from the past. Additionally, it is useful to continuously monitor the prognostic results, to ascertain their plausibility and to calibrate the inputs in the previous steps, if improvement potential is identified.
Step 7: Using the prognoses
Now you are ready to use the Prognostic foresight for improved operational and commercial decision making, thus taking a big step in your IoT journey. The newly gained transparent information about future risk profiles will help to improve operations and maintenance. For example, one can improve the maintenance scheduling and begin to migrate from preventive to truly condition-based maintenance schedules.
Useful questions to ask at this stage include:
- How can I adjust my schedule in such a way that I execute maintenance when the equipment condition makes it really necessary?
- How can I use the foresight from Prognostics to avoid too early maintenance interventions?
- How can I better link the maintenance schedule to my production plan?
Now you are ready to benefit from your prognostic efforts
By applying the 7 steps, you will gain a deeper understanding of your equipment and you will probably come across opportunities to technically optimize your condition monitoring. After having gained some experience in handling Prognostics you will soon be able to convert most of your unscheduled downtime into scheduled maintenance. In the mid to long run Prognostics enables you to create commercially optimal condition-based maintenance schedules.
Moritz von Plate is the CEO of Cassantec. Prior to this role, he was CFO of Solarlite and Principal at the Boston Consulting Group. Moritz is an Agricultural Engineer and MBA from Georgetown University
Have an opinion? Join the discussion in our LinkedIn group
Did you know that predictive analytics does not tell you when a product will fail?
Click here if you have an opinion on this podcast or want to see the opinion of others